Chapter 10 Knowing where but not what
Imagine a friend at a museum mentioning that they are trying to keep track of their family members. What do they mean by that? They might mean that they are continuously aware of where each of their children are, and their spouse. They probably also mean that they are keeping track of which of them is where. The laboratory MOT task, however, does not assess participants’ awareness of which target is where — participants report where the targets are, but do not indicate which is which.
This illustrates that there are two important questions about the role of object identities in tracking. The first is how the position updating aspect of tracking works - does it use differences between the distractors’ and targets’ features to help keep track of the targets? The second question is the extent to which the features of targets are available to conscious awareness - do we know what we are tracking?
10.1 The first question: Does position updating benefit from differences in object identities?
10.1.1 Motion correspondence
In industrial settings, algorithms track objects to detect intrusions and threats to safety. In sports, tracking algorithms analyze how the players on a team move relative to each other, and in animal labs they monitor the movements of study subjects. Engineers who develop such algorithms do not confine themselves to using only the locations and motions of objects - they also use the appearance of those objects, for example their shapes and colors. This facilitates matching objects across video frames (known as the correspondence problem, or in engineering as the “data association problem”) (Yilmaz, Javed, and Shah 2006).
The fact that object features are useful for tracking does not necessarily mean that the brain uses them. The division of cortical visual processing into two streams, dorsal and ventral, hints that it might not. The dorsal “where” pathway specializes in motion and position processing, leaving much of object recognition to the ventral stream (Goodale and Milner 1992). This division may help explain why position updating does not seem to involve much processing of objects’ other features.
The Gestalt psychologist Max Wertheimer found that apparent motion was equally strong whether the objects in successive frames had different features or identical features (Wertheimer 1912). Later studies found that featural similarity has some effect, but still only a small one (Kolers and Pomerantz 1971; Burt and Sperling 1981), so the dominant view today is that the visual system does not use feature similarity for motion correspondence to update a moving object’s position. Some caution is appropriate, however, because when the successive presented frames of an object touch or overlap with each other rather than being presented in non-contiguous locations, the results can be different. In such “line motion” or “transformational apparent motion” displays, feature similarity, especially contour continuity, but also color, can determine which tokens are linked together perceptually (Faubert and Von Grunau 1995; Tse, Cavanagh, and Nakayama 1998). Thus, feature similarity can be involved in motion processing, even though in many situations motion correspondence is instead almost completely determined by spatiotemporal luminance patterns. An important characteristic of this process that does does not seem to have been studied is whether the more complex cues documented by Tse, Cavanagh, and Nakayama (1998) and others are processed in parallel. Short-range spatiotemporal luminance relationships (“motion energy”, roughly) are processed in parallel, by local detectors, yielding parallel visual search for a target moving in an odd direction defined by small-displacement apparent motion (T. Horowitz and Treisman 1994). I am not aware of any studies that have investigated this for transformational apparent motion, in a situation where the perceived motion direction is determined by feature similarity . Thus, the possibility remains that feature similarity effects are driven by a capacity-one process, what I have called System B (6).
10.1.2 Feature differences, but not feature conjunction differences, benefit tracking
While motion correspondence is usually driven only by spatiotemporal luminance information, bject featural information can benefit position tracking via the action of feature attention. Attention can select stimulus representations by their color, so that one can, for example, enhance the selection of all red objects in the visual field. Makovski and Jiang (2009) confirmed that this process can benefit MOT. They used eight moving objects, four of which were targets. MOT performance was better when the eight objects were different in color than when they were identical. This was also true when the objects were all different in shape.
Apart from the usefulness of attending to an individual feature when the targets differ in that feature from the distractors, do feature differences otherwise benefit tracking? A large body of evidence has supported Treisman’s theory that feature pairing information, in contrast to individual features, does not efficiently guide attention to targets (A. Treisman and Gelade 1980; Wolfe 2021). Consistent with this, Makovski and Jiang (2009) found that targets having unique feature pairings do not benefit tracking performance. In their “feature conjunction” condition, each object had a unique pair of features, while it shared the individual features with at least one other object. Performance was no better in this condition than if the objects were all identical. It is this pairing situation that prevents featural attention from contributing, and the results suggest that the tracking process itself does not use featural differences.
10.2 The second question: Are we aware of the identities and features of objects we are tracking?
10.2.1 Feature updating
A common view among lay people may be that we are simultaneously aware of the identities of all the objects in the central portion of our visual field, so unless an object actually disappears, hides behind something or someone, or moves to the edge of our visual field, we should always know where everything in the scene is, and we should readily detect any changes to these objects.
Change blindness demonstrations expose how impoverished our ability to detect changes is. They typically use stationary objects, and associated experiments indicate that although people cannot simultaneously monitor a large number of objects for change, they are able to monitor several, perhaps four or five (Rensink 2000). People seem to do this by loading selected objects into working memory and then, in the second frame of a change blindness display, checking whether any are different than what is held in memory..
The ability to load into memory the features of objects for storage and subsequently compare them to a new display with the objects in the same location may have different demands than continuously updating awareness of the changing features of objects. It appears that hundreds of milliseconds are needed to encode several objects into memory (Vogel, Woodman, and Luck 2006; Ngiam et al. 2019). Without a visual transient to call attention to the site of a change, then, the brain is easily overwhelmed by the task of updating the features of the objects in a typical scene. This is even more true of scenes with moving objects, because motion means continuous transients, masking the transient caused by a featural change.
An example of the failure to detect changes to even a limited number of moving objects was provided by Jun Saiki (2002), who had participants view a circular array of colored discs that rotated about the center of the screen. Occasionally discs swapped color when they briefly went behind occluders, and the participants’ task was to detect these color switches. Performance decreased rapidly with disc speed and number of discs, even though the motion was completely predictable, and Jun Saiki (2002) concluded that “even completely predictable motion severely reduces our capacity of object representations, from four to only one or two.” Because we now understand that simple MOT does not work well across occluders, however, that interpretation of the study is limited by the absence of an MOT-type control. However, the finding was taken further by J. Saiki and Holcombe (2012) without occluders, using a field of 200 moving dots. In one condition, half were green and half were red and the task was to detect a sudden change in color of all the dots. Even when all 200 dots simultaneously switched color between red and green, performance in detecting the switch was very poor. Why had such a dramatic change blindness phenomenon never been noticed before? The phenomenon only occurred when the relative proportion of the two colors was approximately the same before and after the switch, indicating that what is sometimes called “summary statistics” for the overall display are updated readily, but individual pairings of dots with colors are not. This phenomenon was made into an even more dramatic demonstration by Suchow and Alvarez (2011). A full explanation of the phenomenon continues to be debated, but I think it makes it clear that non-position feature updating is less likely to occur with a moving object than with stationary objects. For a stationary object, a feature change will typically stimulate motion / transient detectors, drawing attention to the change and triggering an update. Not so with moving objects, as the motion detectors are continually stimulated, so a feature change does not yield an attention-drawing transient.
Even when objects are stationary, featural updating can be slow. Howard and Holcombe (2008) investigated feature updating by having Gabor targets continually change in orientation or spatial frequency. After a random interval of this continuous change, all the objects disappeared and the location of one was cued - the task was to report its last feature value. Participants tended to report an earlier feature value for the object than its value on the last frame, as one would expect due to either a feature integration time or intermittent updating. What was more interesting was that this lag increased with the number of objects monitored. In the spatial frequency condition, the lag was approximately 140 ms when monitoring one Gabor, 210 ms for tracking two Gabors, and 250 ms for tracking four Gabors. The lags increased also for monitoring orientation and for monitoring location, although not nearly as much: 1 orientation = no measurable lag, 2 orientations = 10 ms, and four orientations = 40 ms, and for position 40 ms, 50 ms, and 90 ms. It’s possible that a position cue, which may be updated more in parallel, contributed to the orientation reporting. If the objects were put in motion, it is possible that performance and the effect of target load would be even worse, but to my knowledge this has never been investigated. The results of some other behavioral paradigms also point to feature updating being sluggish (A. O. Holcombe 2009; Callahan-Flintoft, Holcombe, and Wyble 2020).
Overall, these results suggest a very limited-capacity system is required for updating some features, whereas position updating seems less capacity-constrained. One might hope that even with a very limited-capacity system of feature updating, however, that simple maintenance of the features of objects as they move could easily be done. Instead, maintenance of tracked targets’ identities can be very poor, as we will see in the next section.
10.2.2 Maintenance of target features and identities
According to Zenon Pylyshyn’s FINST (Fingers of Instantiation) theory of tracking, a small set of discrete pointers are allocated to tracked targets. Pylyshyn’s idea was that a pointer allows other mental processes to individuate and link up with an object representation, and the pointer’s continued assignment to a target facilitates representing the corresponding object as the same persisting individual (Zenon Pylyshyn 1989). This implies that when tracking multiple targets, people should know which target is which. However, when Pylyshyn tested this, the results turned out differently than he expected. The first of two papers he wrote on the topic was entitled “Some puzzling findings in multiple object tracking: I. Tracking without keeping track of object identities”. Targets were assigned identities either by giving them names or by giving them distinct and recognizable starting positions: the four corners of the screen (Zenon Pylyshyn 2004). Participants were given the standard task of indicating which objects had been designated as targets, but also were asked about the identity of the target - which one it was. Accuracy at identifying which target was which was very low, even when accuracy reporting their positions was high. However, the task was always to report all the locations first and report the identities second, raising the possibility that the need to remember the identities for longer could have contributed to the poorer performance.
More evidence for a disconnect between knowledge of what one is tracking and success at the basic MOT task was found by T. S. Horowitz et al. (2007), who had participants track targets with unique appearances - the stimuli were cartoon animals in one set of experiments. At the end of each trial, the targets moved behind occluders so that their identities were no longer visible. Participants were asked where a particular target (say, the cartoon rabbit) had gone - that is, which occluder it was hiding behind. This type of task had been dubbed “multiple identity tracking” by Oksama and Hyönä (2004). Performance was better than chance, but was worse than the standard MOT task of reporting target locations irrespective of which target a location belonged to. This basic finding was replicated in four additional experiments. The effective number of objects tracked, as reflected in a standard MOT question, was around three or four, but for responses about the final location of a particular animal, capacity was estimated as closer to two objects. So, the evidence seems robust that knowledge of which target is which is often poor, in contrast to Pylyshyn’s original view that this information was part and parcel of the tracking mechanism.
A counterpoint is that Wu and Wolfe (2018) found only a fairly small performance deficit for identity reporting relative to position reporting. Using MOT and MIT tasks carefully designed to be comparable, they had participants track 3, 4, or 5 targets, and found 96%, 89%, and 86% accuracy for the MOT task, against 93%, 85%, and 79% accuracy for the MIT task. While the high performance for the 3-target condition could be a ceiling effect, that is probably not the case for the 4- and 5-target conditions. One difference with previous work is that at the beginning of a trial, all the stimuli (cartoon animals) were stationary and participants had unlimited time to memorize the targets’ locations. When a participant was ready, they would press a key and the animals transformed into identical gray circles and began moving. At the end of the trial, one of the circles was probed and the participant either had to indicate whether it was a target, or indicate whether it had originally been a particular animal. One possible explanation of the discrepancy with previous findings is that while identities are not native to tracking’s pointers in the way Pylyshyn thought, with adequate time for memorization the associations can be made and maintained.
10.2.3 Beaten by a bird brain
Pailian et al. (2020) investigated identity maintenance during tracking in a format like a hustler’s shell game. The engaging nature of the shell game format made it suitable for testing children and an African grey parrot as well as human adults.
As stimuli, Pailian et al. (2020) used colored wool; real balls of wool, actually, not pictures on a screen. Between one and four of the balls were shown to a participant, after which the experimenter covered the balls with inverted plastic cups, and with their hands swapped the positions of first one pair, then another. After a variable number of swaps, the experimenter produced a probe ball of one of the target colors, and the task was to point to (or peck on, in the case of the parrot), the cup containing the probed color.
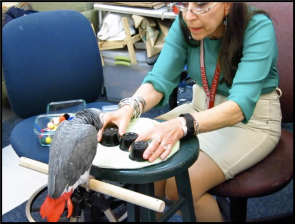
Figure 12: An African grey parrot participates in the shell game used by Pailian et al. (2020). CC-BY Hrag Pailian.
I would have predicted that people would be able to perform this task with high accuracy, especially given that not only were only two objects in motion at any one time, the experimenter paused for a full second between swaps, which ought to give people sufficient time to update their memory of the locations of those two colors. When only two balls were used, accuracy was in fact high: over 95%, even for four swaps, which was the highest number tested. This was true for the human adults, the parrot, and the 6- to 8-year-old children alike.
In the three-ball condition, the adults did fine when there were only a few swaps, but their performance fell substantially as the number of swaps increased, to about 80% correct for four swaps. For some reason, participants did not reliably update the colors for four swaps. The effect of number of swaps was more dramatic for the children. They performed near ceiling for the zero-swap (no movement) condition, but accuracy fell to close to 80% in the one-swap condition, and to around 70% for two and three swaps.
Remarkably, the parrot actually outperformed not only the children, but also the human adults. It seems that this was not due to more practice - the authors state that the parrot learned the task primarily by simply viewing the experimenter and a confederate perform three example trials (the parrot was experienced with a simpler version of the task involving only one object presented under one of the three cups). That the bird had the ability to remember and update small sets of moving hidden objects to a level of accuracy similar to humans, despite having a brain less than one-fiftieth the size of ours, is remarkable.
What needs to be explained is why the Harvard undergraduates, who almost surely had above-average intelligence and motivation, displayed levels of accuracy that were not very high for when there were more than a few swaps. Prior to the publication of this study, I had assumed that the reason for poor performance in multiple identity tracking was the difficulty of updating the identity of three or four targets simultaneously while they moved. I would have predicted that changing positions exclusively by swapping the positions of just two objects, with a one-second pause between swaps, would keep performance very high. These results, then, suggest that updating the memory of object locations is quite demanding. Thus, not only does identity updating not happen automatically as a result of object tracking, but also it may rely on a very sluggish memory updating system.
Another reason many should be surprised is the popularity of the “object files” idea that all the features of an object are associated with a representation in memory, the object file, that is maintained even as an object moves (Kahneman, Treisman, and Gibbs 1992). In associated experiments, a trial begins with a preview display with two rectangles. Each rectangle contains a feature - usually a letter. The fletter disappears, and the rectangles move to a new location. The observer’s representation of the display is then probed by presenting a letter once again in one of the rectangles, or elsewhere, and asking participants to identify it. If the letter is the same as the one presented in that rectangle at the beginning of the display, observers are faster to respond than if it had appeared in another rectangle in the beginning of the display, indicating that that aspect of the rectangle’s initial properties was maintained, with its location updated. One difficulty with interpreting this response time priming phenomenon is that, because responses must be averaged over many trials to reveal it, we do not know on what proportion of trials it is effective. Thus it is hard to know whether it is inconsistent with the behavioral findings mentioned above that show successful updating on only a minority of trials.
There is also the question of the capacity of the object-file system: whether several object files could easily be maintained and updated. Kahneman, Treisman, and Gibbs (1992) found that the amount of priming was greatly diminished when four letters were initially presented in different rectangles, suggesting that fewer objects than that had letter information maintained and updated. They concluded that there may be a severe capacity limit on object files or object file updating. The evidence from the studies in this chapter overall suggests that identity updating is very poor in a range of circumstances.
10.2.4 Some dissociations between identity and location processing reflect poor visibility in the periphery
To explain why participants don’t update the identities of tracked moving objects nearly as well as they update their positions, the Finnish researchers Lauri Oksama and Jukka Hyönä suggested that identities are updated by a serial one-by-one process, while positions are updated in parallel. Oksama & Hyönä were motivated by evidence from eye tracking. During an MIT task, Oksama and Hyönä (2016) found that participants frequently looked directly at targets, for more than 50% of the trial duration, and frequently moved their eyes from one target to another. In contrast, during MOT, the participants moved their eyes infrequently, and their gaze wasn’t usually at any of the moving objects, rather they were more often looking somewhere close to the center of the screen. Oksama and Hyönä (2016) took these results to mean that the targets’ identity-location bindings that must be updated during MIT are updated by a serial one-by-one process, whereas target positions during MOT are updated by a parallel process; for a review, see Hyönä, Li, and Oksama (2019).
A problem for interpreting the Oksama and Hyönä (2016) results is that participants may have had to update target identity information one-by-one purely due to limitations on their peripheral vision. That is, the targets (line drawings) likely were difficult to identify when in the periphery. Thus, participants may have had to move their eyes to each object to refresh their representation of which was which. Indeed, in a subsequent study, Li, Oksama, and Hyönä (2019) tested discriminability of the objects in the periphery and found that accuracy was poor. When colored discs were used as stimuli instead of line drawings, accuracy was higher in the periphery and participants did not move their eyes as often to individual targets, which suggests at least some degree of parallel processing, leaving the amount of serial processing for simple colors, if any, in doubt.
Many findings of differences between MIT and MOT performance may be explained by poor recognition of the targets in the periphery. One could blur the objects to impair localization but it is not clear what degree of spatial uncertainty is comparable to a particular level of object identifiability; an apples-and-oranges problem.
One dissociation between identity and location tracking performance seems to remain valid regardless of the difficulty of perceiving object identities in the periphery. This is the original finding by Zenon Pylyshyn (1989), replicated by M. Cohen et al. (2011), that if targets are actually identical but are assigned different nominal identities, participants are very poor at knowing which is which at the end of the trial because in this paradigm, there is no visible identity information.
10.2.5 Evidence from two techniques suggests parallel updating of identities
Piers D. L. Howe and Ferguson (2015) used two techniques to investigate the possibility that serial processes are involved in multiple identity tracking. First, Howe et al. applied a simultaneous-sequential presentation technique that when applied to MOT had yielded evidence for no serial processing (Piers D. L. Howe, Cohen, and Horowitz 2010). In the technique, the stimuli are presented either all at once (simultaneously) or in succession (sequentially). In the successive condition, half the stimuli were presented in the first interval of a trial, and the other half in the second interval. If a serial process is required to process each stimulus, the prediction is that performance should be better in the sequential condition, as the presentation duration of each stimulus is equated for the simultaneous and successive conditions, but in the simultaneous condition a one-by-one process wouldn’t have enough time to get through all the stimuli. The technique has been applied extensively to the detection of a particular briefly-presented alphanumeric character among other briefly-presented alphanumeric characters, and researchers have found that processing in the simultaneous condition is equal to or better than the sequential condition, suggesting that at least four alphanumeric characters can be recognized in parallel (Shiffrin and Gardner 1972; Hung et al. 1995).
As a MIT simultaneous-sequential paradigm, Piers D. L. Howe and Ferguson (2015) presented four targets of different colors moving among four distractors. Each of the four distractors was the same color as one of the targets, so that the targets overall could not be distinguished from the distractors by color. In the simultaneous condition, all the objects moved for 500 ms and then paused for 500 ms, a cycle that repeated throughout the trial. In the sequential condition’s cycle, half the targets moved for 500 ms while the other half were stationary, and subsequently the other half of targets moved for 500 ms while the others remained stationary. Performance was similar in the simultaneous and sequential conditions, supporting the conclusion that there was no serial process required for the task (Piers D. L. Howe and Ferguson 2015). This conclusion is limited, however, by an assumption that any serial process could respond efficiently to the movement cessation of half the targets by shifting its resources to the moving targets, while not causing any forgetting of the locations and identities of the temporarily-stationary targets. To support this assumption, Piers D. L. Howe and Ferguson (2015) pointed out that Hogendoorn, Carlson, and Verstraten (2007) had shown that attention could move at much faster rates than 500 ms per shift. However, the Hogendoorn, Carlson, and Verstraten (2007) studies did not assess the attention shifting time between unrelated targets, rather their shifts were for attention stepping along with a single target disc as it moved about a circular array. Thus, it is unclear how much the results of Piers D. L. Howe and Ferguson (2015) undermine the serial, one-by-one identity updating idea embedded in the theories of Oksama & Hyönä and Lovett, Bridewell, and Bello (2019).
Piers D. L. Howe and Ferguson (2015) further investigated serial versus parallel processing in MIT by using another technique: systems factorial technology (Townsend 1990). The two targets were presented in the same hemifield, to avoid independence by virtue of the hemispheres’ independence (G. A. Alvarez and Cavanagh 2005). The participants were told to monitor both moving targets and that if either darkened, to press the response button as quickly as possible, after which all the disks stopped moving and the participant was asked to identify the location of a particular target, for example the green one (the objects were identical during the movement phase of the trial but initially each was shown in a particular color). To ensure that participants performed the identity tracking task as well, only trials in which the participant reported the target identity correctly were included in the subsequent analysis. Detection of the darkening events was very accurate (95% correct). On different trials, either both targets darkened, one of them darkened, or neither of them darkened, and each could darken either by a small amount or by a large amount. The pattern of the distributions of response time for the various conditions ruled out serial processing (if one accepts certain assumptions), implicating limited-capacity parallel processing. This suggests that participants can process luminance changes of two moving targets in parallel while also maintaining knowledge of the identity of the moving targets. One reservation, however, is that it is unclear how often the participants needed to update the target locations and refresh their identities, because the rate at which they needed to be sampled to solve the correspondence problem is unclear for the particular trajectories used (this issue is explained in Alex O. Holcombe (2022)). It also would be good to see these techniques applied to targets defined only by distinct feature conjunctions, with no differences in features between the targets and the distractors. This would prevent any contribution of feature attention, and with processing of feature pairs likely to be more limited-capacity than that of identifying individual features, the results might provide less evidence for parallel processing.
10.3 Eye movements can add a serial component to tracking
Partially in response to the evidence of Piers D. L. Howe and Ferguson (2015) against serial processing in tracking, Oksama and Hyona, with Jie Li, revised their Model of Multiple Identity Tracking (MOMIT) to add more parallel processing. MOMIT 2.0 states that the “outputs of parallel processing are not non-indexed locations but proto-objects that contain both location and basic featural information, which can be sufficient for tracking in case no detailed information is required” (Li, Oksama, and Hyönä 2019). This is a reasonable response to the evidence, even if it unfortunately means the theory doesn’t make as strong predictions, as the role of serial processing is now more vague. In this model, serial processing is tied to eye movements and is used to acquire detailed visual information for refreshing working memory representations. The theory seems to be mute on whether serial processing would be involved if both fixation were enforced and the stimuli were easily identifiable in the periphery.
Let’s step back and consider the role of eye movements in everyday behavior. People move their eyes about three times a second, partly because it is usually adaptive to direct the fovea at whatever object we are most interested in. Rarely are all visual signals of interest clustered together enough that they can be processed adequately without moving the fovea among them. Finally, animals like ourselves have drives to explore visual scenes, because we evolved in complex and changing environments. Perhaps, then, one should expect frequent eye movements to occur even when they are not strictly necessary.
Eye movements usually contribute a serial, one-by-one component to processing, because high-resolution information comes from only a single region on the screen - the region falling on the fovea. People are cognitively lazy in that they seem to structure eye movements and other actions in tasks so as to minimize short term memory requirements (Hayhoe, Bensinger, and Ballard 1998). Even when saccading to different targets is inefficient because people can keep information in memory, and update information in the periphery, people may move their eyes anyway. The most interesting evidence for serial processing, then, may be that found when eye movements are prohibited. The steep decrease with load of apparent sampling frequency discovered by Alex O. Holcombe and Chen (2013) provides some evidence for that.
To summarise this Section, both use of object identities in tracking and the updating of target identities for awareness is typically poor. This fits with broader findings over the last thirty years that the mind maintains fewer explicit visual representations than we intuitively believe but quick attentional deployment to tracked locations means that the world can serve as an outside memory for content (O’Regan 1992).